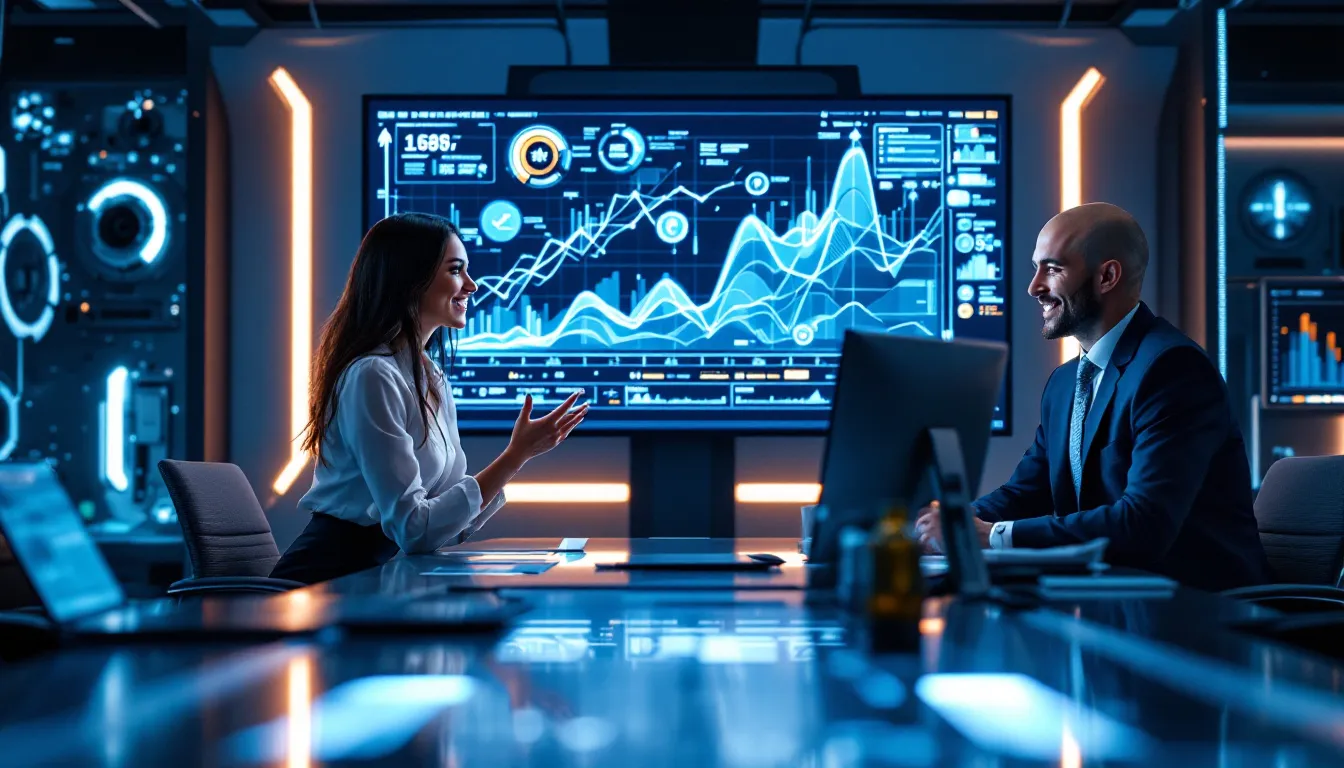
AI for Seasonal Demand Forecasting: Guide 2024
AI is revolutionizing seasonal demand forecasting. Here’s what you need to know:
- AI crunches massive data sets to find hidden patterns
- It adapts to market changes faster than traditional methods
- Businesses use AI forecasting to stay competitive
Key benefits:
- Less waste
- Fewer stockouts
- Happier customers
How AI improves forecasting:
- Analyzes historical sales, product info, and external factors
- Uses machine learning to spot trends humans might miss
- Updates predictions in real-time as new data comes in
To get started with AI forecasting:
- Clean and prepare your data
- Choose the right AI model for your needs
- Train and test your model
- Monitor and adjust regularly
Remember: AI isn’t perfect. It needs good data and human oversight to work well.
Quick Comparison: AI vs Traditional Forecasting
Feature | AI Forecasting | Traditional Forecasting |
---|---|---|
Data handling | Many variables | Few variables |
Adaptability | Auto-learning | Static |
Accuracy | More accurate | Less accurate |
Speed | Fast | Slow |
Cost savings | Up to 75% inventory cost reduction | Limited |
AI forecasting is powerful, but it’s not magic. Use it wisely, and you’ll navigate seasonal demand like a pro.
Related video from YouTube
What is Seasonal Demand?
Seasonal demand is when people buy more (or less) of something at certain times of the year. It’s like clockwork – you can predict it.
Why Does It Happen?
A few reasons:
- Weather: Think swimsuits in summer, coats in winter.
- Holidays: Christmas trees in December, anyone?
- Payday: Some folks shop more when they get paid.
- Industry stuff: Tax pros are swamped from January to April.
The Tricky Part
Predicting seasonal demand isn’t always easy. Here’s why:
1. Bad Data
If your past numbers are off, your predictions will be too.
2. People Change
What worked last year might not work this year.
3. Surprises
A big storm or economic hiccup can mess everything up.
4. New Products
No history = tough to predict.
Knowing these patterns (and problems) helps businesses plan better. It’s all about being ready for the ups and downs.
How AI Helps with Forecasting
AI and ML are revolutionizing seasonal demand forecasting. Here’s how:
AI and Machine Learning Basics
AI crunches massive data in minutes, not weeks. This speed lets businesses pivot fast.
ML models learn from data, improving forecasts over time. They spot patterns humans might miss.
Main AI Methods Used
1. Time Series Analysis
Great for seasonal products. Uses past sales to predict future demand.
2. Machine Learning Models
Handles various factors affecting demand across product ranges.
3. Neural Networks
Finds hidden patterns in complex data, ideal for tricky forecasts.
Benefits Over Old Methods
AI forecasting outperforms traditional methods:
Feature | Traditional | AI |
---|---|---|
Data Handling | Few variables | Many variables |
Adaptability | Static | Auto-learning |
Accuracy | Less accurate | More accurate |
Speed | Slow | Fast |
Real-world impact:
- AI cuts supply chain errors by 30-50% (McKinsey Digital)
- Reduces out-of-stock lost sales by up to 65%
"AI makes this happen at the push of a button!" – Supply Chain Expert
AI transforms business operations:
- Optimizes inventory
- Smooths supply chain
- Frees staff for strategy
H&M uses ML to predict fashion trends, stocking the right clothes in the right stores. This tackles the industry’s $562 billion overstocking problem.
Getting Data Ready for AI Forecasting
AI forecasting needs clean data to work well. Here’s how to prep it:
Needed Data Types
For good AI forecasts, you’ll want:
- Historical sales data
- Product info
- Pricing data
- Inventory levels
- Marketing campaign dates
- External factors (weather, events)
How to Collect and Clean Data
1. Gather data from all sources
Put all your data in one place. This is crucial.
2. Standardize formats
Make everything consistent. For example, use the same currency for all prices.
3. Remove errors and duplicates
Fix things like:
- Typos
- Wrong values
- Duplicate entries
4. Handle missing data
Either remove incomplete records or fill gaps with stats.
5. Validate data quality
Make sure your data makes sense and follows your business rules.
Creating Useful Data Features
Turn raw data into AI-friendly features:
- Time-based: Add columns for day of week, month, season
- Lag features: Include past sales as new columns
- Rolling stats: Add moving averages or totals
Pro tip: Use tools to speed this up. Ikigai‘s aiMatch can merge data sets fast, saving you time.
Step | Why It Matters | Example |
---|---|---|
Consolidation | Get all data in one place | Combine online and in-store sales data |
Standardization | Make formats consistent | Use YYYY-MM-DD for all dates |
Cleaning | Fix errors | Correct product code typos |
Feature Engineering | Create useful new data points | Flag holidays in your data |
Good data prep = better AI forecasts. McKinsey found 75% of supply chain leaders still use spreadsheets. AI can give you an edge, but only with clean data.
Building AI Models for Seasonal Forecasting
Let’s dive into how to build AI models for seasonal demand forecasting:
Steps to Build a Model
- Set a clear goal: What are you predicting? Sales? Website traffic? Inventory needs?
- Get your data: Grab historical sales data, product info, and external factors like weather.
- Clean it up: Fix errors, fill in gaps, and create useful features.
- Pick your model: Choose an AI method that fits your data and goals.
- Train it: Feed your prepared data to the AI.
- Test it: See how well it works on new data.
- Use and watch: Put it to work and keep an eye on it.
Choosing Your AI Method
Your choice depends on your data and needs:
AI Method | Good For | Data Needed |
---|---|---|
Prophet | Strong seasons | 1+ year |
ARIMA | Short-term, stable | Stationary time series |
LSTM | Complex, long-term | Lots of data |
For example, if you’re a retailer with clear seasonal sales, Prophet might be your best bet.
Training and Testing
Here’s how to train your model right:
- Split data: 80% for training, 20% for testing.
- Cross-validate: Ensures it works on new data.
- Tweak settings: Adjust for better results.
- Check accuracy: Use metrics like MAPE or RMSE.
"Cross-validation boosted our forecast accuracy by 40%", – Sarah Chen, Data Scientist at Walmart.
Your model needs to predict the future, not just match past data.
Real-world example: In 2022, Zara used AI to forecast demand. They analyzed social media and user feedback to predict fashion trends, cutting unsold inventory by 20%.
Advanced AI Forecasting Techniques
AI forecasting has evolved. Here are some cutting-edge methods to supercharge your seasonal demand predictions:
Adding Outside Factors
AI models now crunch more than sales data. They factor in:
- Weather patterns
- Economic indicators
- Social media trends
This wider lens catches demand shifts you might miss.
Starbucks uses weather data to tweak drink forecasts. Hot days? More Frappuccinos. Cold snaps? Hot coffee sales jump.
Factor | Forecasting Impact |
---|---|
Weather | Shifts product preferences |
Economic indicators | Affects buying power |
Social media | Signals consumer interests |
Combining Models for Better Results
One model is good. Several can be better. This is ensemble learning:
1. Run multiple AI models on your data
2. Compare their predictions
3. Combine results (often by averaging or voting)
This smooths out individual model quirks, leading to steadier forecasts.
"Companies using predictive analytics boost efficiency by 30%+ and revenues by 5-10%." – McKinsey
Dealing with Long-Term Changes
Seasonal patterns aren’t fixed. They shift due to:
- Changing consumer habits
- New competitors
- Global events
Advanced AI spots these long-term trends and adjusts. This keeps predictions sharp as markets evolve.
Some AI systems now use adaptive learning. They update their understanding with new data, keeping forecasts fresh and relevant.
sbb-itb-178b8fe
Checking and Improving AI Forecasts
AI forecasts are only as good as their accuracy. Here’s how to check and boost your model’s performance:
Key Metrics
Use these to gauge your AI forecast’s effectiveness:
Metric | Measures | Why It’s Important |
---|---|---|
Mean Absolute Error (MAE) | Average forecast deviation | Shows overall accuracy |
Root Mean Square Error (RMSE) | Impact of larger errors | Spots big misses |
Balanced Accuracy | Performance across classes | Vital for uneven data |
Balanced accuracy is crucial for seasonal forecasts. It’s the average of true positive and true negative rates, giving a clear picture across all demand levels.
Boosting Model Performance
1. Clean Your Data
Get rid of duplicates, fix errors, and fill gaps. Better data = better forecasts.
2. Engineer Features
Create new data points for seasonal trends. Flag holidays or events that affect demand.
3. Use Ensemble Learning
Combine multiple models. It smooths out individual quirks.
Fixing Common Issues
Overfitting to Past Seasons? Use cross-validation across multiple years. It helps your model adapt to new patterns.
Missing Sudden Demand Shifts? Add external data like weather or economic indicators. As Guy Peri, Procter & Gamble’s chief data officer, puts it:
"For AI to be successful, we need strong data management practitioners to ensure we have quality, reliable data going into the algorithms so the insights and actions recommended by the algorithms deliver the right outcomes for the business."
Poor Performance on Minority Classes? Use oversampling to balance your dataset. Your model will learn from all demand levels, not just the common ones.
Using AI Forecasts in Business
AI forecasts are changing inventory and supply chain management. Here’s how to use them effectively:
Making Sense of AI Predictions
AI turns data into business insights. To use them:
- Spot patterns in AI output
- Compare forecasts to past results
- Check if predictions match your business know-how
Amazon uses AI to analyze customer data and predict orders, helping them stock warehouses efficiently.
Matching Forecasts with Operations
Once you have AI predictions, act on them:
1. Adjust inventory
Use forecasts to set stock levels. This cuts costs and prevents stockouts.
2. Plan staffing
Schedule workers for predicted busy times.
3. Optimize supply chain
Share forecasts with suppliers to improve ordering.
Walmart uses AI during peak times to manage stock across stores and warehouses, keeping shelves full when customers need items most.
Keeping Forecasts Up-to-Date
To keep AI forecasts accurate:
- Feed new data into your AI system often
- Check forecast accuracy against real results
- Adjust your AI model if it’s off target
Johnson & Johnson updates their AI with new global supply chain data constantly. This helps them respond quickly to demand changes.
Real Examples of AI in Seasonal Forecasting
Industry Success Stories
AI has revolutionized seasonal demand forecasting. Here’s how big players are using it:
1. Walmart’s AI-Driven Supply Chain
Walmart’s AI analyzes store, distribution, and external data to:
- Boost demand prediction accuracy
- Fine-tune inventory
- Cut down on stockouts and overstocks
Result? Lower costs and happier customers.
2. DHL‘s Smart Logistics
DHL’s AI:
- Crunches traffic and weather data
- Optimizes delivery routes
- Speeds up deliveries
- Reduces fuel use
Bonus: A smaller carbon footprint.
3. Procter & Gamble’s Promotion Optimization
P&G uses machine learning to:
- Refine promotions and pricing
- Improve forecasts
- Shape demand patterns
Key Takeaways
1. Data Integration Is Key
Successful AI forecasting mixes:
- Past sales data
- Seasonal trends
- Economic factors
- Competitor moves
- Real-time info
2. AI Beats Traditional Methods
AI forecasts show clear wins:
Metric | Improvement |
---|---|
Forecast Error | Down 30-50% |
Lost Sales | Down 65% |
Inventory Costs | Down 40% |
3. Continuous Learning Matters
Top AI systems adapt to market changes, getting smarter over time.
Best Practices
- Go Beyond Basic Forecasting Use advanced analytics for SKU/store-level optimization.
- Mix Internal and External Data Amazon’s "anticipatory shipping" uses order history, search data, wish lists, and more.
- Think Big Automated data processing makes advanced analytics scalable.
- Connect Your Supply Chain Integrated data across the supply chain is crucial for accurate forecasts.
Problems with AI in Seasonal Forecasting
AI has revolutionized seasonal demand prediction. But it’s not flawless. Here’s a look at some issues and solutions.
Common Mistakes to Avoid
Trusting AI Too Much
AI isn’t omniscient. Daniel, a Senior Demand Planner at Energizer Holdings, cautions:
"All models fall short of representing reality with perfect accuracy."
Don’t rely solely on AI. Blend it with human insight.
Poor Data Quality
Garbage in, garbage out. Ensure your data is clean, current, and diverse.
Ignoring Outside Factors
AI might miss major demand-altering events like global crises, new competitors, or sudden trends. Keep your eyes open.
Not Updating Models
Markets evolve. So should your AI. Regularly update your models to maintain accuracy.
Ethical Issues to Consider
Data Privacy
AI’s data hunger can clash with privacy. Be cautious with customer, employee, and supplier information.
Fairness in Decision-Making
AI can be biased, leading to unfair choices about suppliers, stock distribution, or pricing. Regular bias checks are crucial.
Job Displacement
AI might replace some roles. Plan ahead: consider retraining staff, creating new positions, or using AI as a tool, not a replacement.
What’s Next for AI Forecasting
Better Integration
Future AI will mesh seamlessly with other systems, enabling quicker responses, more accurate predictions, and easier use for non-experts.
More Data Sources
AI will tap into diverse data streams like social media trends, weather patterns, and economic indicators, boosting forecast accuracy.
Explainable AI
Upcoming AI will be more transparent, fostering trust, facilitating quick error correction, and meeting regulatory requirements.
Conclusion
AI has shaken up seasonal demand forecasting. It’s not perfect, but it’s a game-changer for accuracy and speed.
Here’s the deal:
- AI crunches massive data sets, finding patterns we’d miss
- It adapts to market changes faster than old-school methods
- Businesses are jumping on AI forecasting to stay ahead
The perks? Less waste, fewer stockouts, and happier customers.
But here’s the catch: AI needs work to shine.
- You need clean, solid data
- Models need regular updates
- Humans still need to keep an eye on things
What’s next for AI forecasting?
- Smoother integration with other business tools
- More diverse data sources
- Clearer explanations of its decisions
Thinking about AI forecasting? Remember:
- Set clear goals and clean up your data
- Pick AI methods that fit YOUR business
- Keep checking AI forecasts against real results
AI isn’t magic. But use it right, and you’ll navigate seasonal demand like a pro.
FAQs
How AI can forecast demand in supply chain?
AI is changing the game in supply chain demand forecasting. Here’s how:
1. Slashing errors
AI-based forecasting cuts errors by 20-50%. This means:
- 65% fewer lost sales
- 65% less product unavailability
2. Smarter inventory
AI helps companies:
- Cut warehousing costs by 5-10%
- Reduce admin expenses by 25-40%
3. Boosting accuracy
AI crunches massive datasets, considering multiple factors for better predictions.
Check out how top companies are using AI:
Company | AI Use | Outcome |
---|---|---|
Zara | Analyzes user feedback and social media | Better products and targeted marketing |
Amazon | Tracks competitor prices and market trends in real-time | Optimized pricing across global network |
Siemens | Studies market trends and past sales | Improved production schedules |
Tesla | AI-powered demand forecasting | Better production efficiency and order fulfillment |
"AI-based forecasting transforms supply chain management by reducing errors by 20-50%. This translates into cutting lost sales and product unavailability by up to 65%." – McKinsey
AI in supply chain forecasting delivers:
- Real-time insights
- Happier customers
- More profit
- Smoother operations