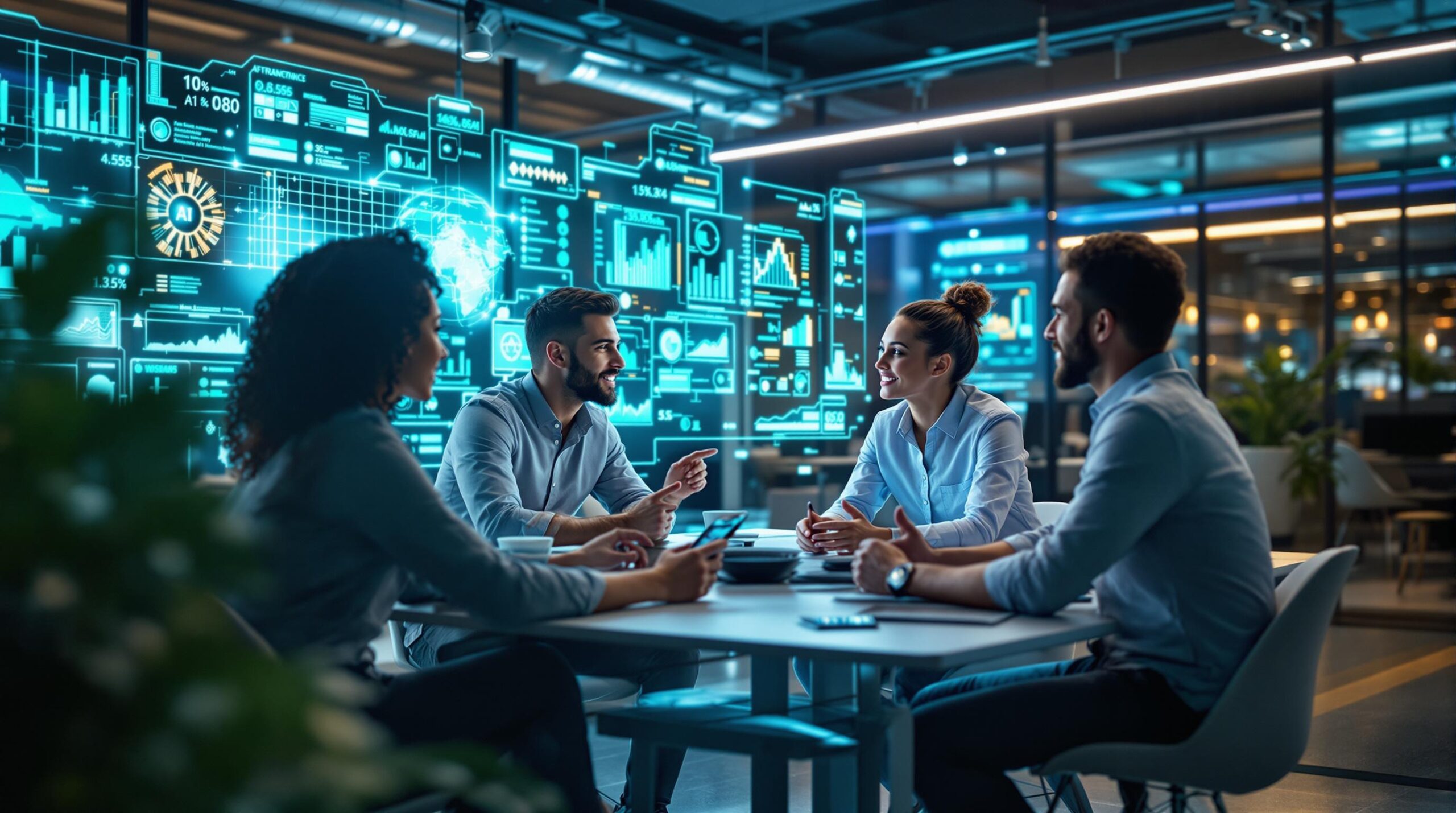
Dynamic Process Adaptation in AI Automation
Dynamic process adaptation lets AI adjust workflows in real time, improving efficiency and flexibility for businesses. Unlike traditional processes that rely on fixed rules and manual updates, this approach uses AI and machine learning to continuously optimize decision-making and operations.
Key Takeaways:
- Real-Time Adjustments: AI adapts workflows instantly based on live data.
- Improved Efficiency: Reduces downtime, errors, and manual intervention.
- Scalable Solutions: Tools like EverEfficientAI and Aisera customize workflows to unique business needs.
- Applications Across Industries: From customer service to supply chain management, dynamic AI is transforming operations.
- Challenges to Address: Data quality, system integration, and ethical concerns like bias and privacy.
Quick Comparison: Traditional vs. Dynamic Process Adaptation
Feature | Traditional Processes | Dynamic Process Adaptation |
---|---|---|
Response Time | Days or weeks | Real-time adjustments |
Process Updates | Manual reviews required | Continuous AI-driven optimization |
Scalability | Limited | Highly scalable and customizable |
By leveraging AI-powered tools and focusing on high-quality data, businesses can streamline operations and stay competitive in rapidly changing markets.
Business Process Analysis
Core Elements of Dynamic Process Adaptation
AI and Real-Time Data Analysis
AI systems today analyze data streams as they come in, reshaping how businesses manage ever-changing processes. These systems handle multiple data sources at once – like sensor data or customer interactions – allowing businesses to respond quickly to shifting conditions.
This real-time analysis minimizes downtime, makes better use of resources, and improves quality control. For example, businesses have reported 35-45% fewer equipment failures and a 30% drop in production defects. These stats show how real-time data analysis can lead to better operations across various sectors.
However, the real magic happens when machine learning steps in to interpret this data and make decisions.
Decision-Making with Machine Learning
Machine learning plays a key role in dynamic process adaptation by enabling systems to make decisions based on context. These AI systems can adjust in real time, learning from new data to continuously improve their decision-making.
Techniques like online learning, transfer learning, and active learning allow these systems to adapt and refine their processes. For machine learning to fully transform business operations, it needs to be both scalable and customizable to fit specific needs.
Scalable and Tailored Solutions
Companies like EverEfficientAI and Aisera are paving the way with solutions that scale and adapt to unique business requirements. EverEfficientAI offers AI-powered dashboards that adjust to individual needs, while Aisera’s Dynamic Action Flows streamline complex processes through automation [2]. These examples show how adaptable solutions can address specific challenges businesses face.
To scale effectively, these systems need flexible designs, smooth integration with current tools, and workflows tailored to the industry they serve.
Steps to Implement Dynamic Process Adaptation
Choosing the Best AI Tools
When picking AI platforms, look for ones that offer flexible automation and easy integration. Prioritize tools with features like customizable workflows, strong monitoring capabilities, and the ability to scale with your needs. Integration options are also key – your tools should fit seamlessly into your existing systems.
Once you’ve chosen your tools, explore real-world use cases to better understand how they can be applied to your specific needs.
Real-World Success Stories
Dynamic process adaptation is changing the game in various industries.
For example, in customer service, AI-powered chatbots now handle routine inquiries while forwarding complex questions to human agents. This setup has drastically improved response times and boosted customer satisfaction [1].
In HR, AI has delivered impressive results – cutting document processing times by 65%, reducing manual errors by 80%, and speeding up onboarding processes by 40%. These examples show how effective dynamic process adaptation can be, but getting it right requires thoughtful planning and execution.
Practical Tips for Implementation
Start small with pilot projects to test how AI integrates into your workflow. This approach helps spot issues early before scaling up.
Here are some key areas to focus on:
- Data Management: High-quality, relevant data is essential for AI accuracy. Use strong data validation processes and schedule regular quality checks to keep your systems reliable.
- Team Training: Equip your team with the skills to use AI tools effectively. Training on both the tools and data analysis will encourage smoother adoption.
- Ongoing Monitoring: Use detailed logs and dashboards to track performance metrics. Regular reviews will help you align AI systems with your business goals and keep them running at their best.
sbb-itb-178b8fe
Challenges and Things to Keep in Mind
Technical Challenges
Bringing dynamic process adaptation into an organization isn’t without its hurdles. One of the biggest challenges? Data quality. Poor-quality data can lead to inaccurate AI predictions and costly mistakes, derailing efficiency and decision-making.
Another sticking point is system integration. Combining AI with older legacy systems, managing APIs, and maintaining performance at scale takes careful planning and execution. It’s no small task.
Then there’s the complexity of training AI models. This can become especially tricky when dealing with business processes that are highly variable or intricate.
But the challenges don’t stop at technology. Organizations also need to think about the ethical and human side of AI-driven processes.
Ethical and Workforce Concerns
When adopting dynamic process adaptation, ethics should be front and center. Data privacy is a growing concern, especially as AI systems handle more sensitive information. Staying compliant with data protection laws and maintaining transparency is non-negotiable.
Another issue? Algorithmic bias. Bias can creep in when datasets aren’t diverse enough or when AI systems aren’t regularly audited. Tackling this requires diverse data, frequent checks, and clear development practices.
Then there’s the workforce. While AI can make processes faster and more efficient, it also stirs up fears about job displacement. The solution? Retraining employees to take on roles where they manage and optimize AI systems.
Take Aisera’s use of Dynamic Action Flows, for example. They’ve shown how businesses can balance precision, speed, and scalability while keeping ethical considerations in mind [2].
These challenges might seem daunting, but addressing them head-on is critical for getting the most out of dynamic process adaptation.
What’s Next for Dynamic Process Adaptation
New Trends in AI Automation
Advances in Natural Language Processing (NLP), predictive analytics, and real-time machine learning are reshaping AI automation. For example, NLP enables more refined interactions between humans and machines. This is especially useful in customer service, where AI-driven chatbots can now handle complex queries with ease.
Real-time machine learning has taken data analysis to the next level. It can predict risks and take preventive actions before issues escalate, especially in AI-enhanced phases and gates processes [1].
"AI integration plays a crucial role in intelligent automation. It involves embedding AI capabilities within existing automation tools and processes to enhance their functionality and intelligence." – Enhanced.ai [3]
Predictive analytics has also become a game-changer, helping businesses forecast market trends, customer behavior, and operational inefficiencies. This is transforming decision-making in industries with complex challenges.
These developments aren’t just improving current processes – they’re opening up entirely new ways to use AI in business.
Expanding Applications of AI
AI is being applied in ways that go far beyond traditional automation. Businesses are finding novel uses for AI across different functions:
Application Area | Example | Impact |
---|---|---|
Customer Service | Sentiment analysis tools | Faster responses, happier customers |
Operations | Predictive maintenance | Less downtime, lower costs |
Marketing | Real-time campaign adjustments | Better engagement, higher ROI |
Supply Chain | Smarter inventory systems | Reduced waste, optimized stock levels |
Take EverEfficientAI as an example. Their predictive analytics tools and dynamic dashboards allow businesses to adapt processes in real time, keeping them competitive in fast-changing markets.
Hybrid workflows are another exciting development. These combine AI’s analytical power with human expertise – AI handles the data crunching, while people focus on big-picture strategy. Instead of replacing jobs, AI is enhancing how decisions are made.
AI is also breaking new ground by analyzing unstructured data from multiple sources. This allows it to detect patterns and trends that humans might miss. Integration with platforms like Zapier and Make is further streamlining processes, connecting systems, and enabling smoother workflows across businesses.
Summary and Final Thoughts
Key Points and Strategies
Dynamic process adaptation is changing the way businesses operate in the AI era. Recent statistics show that 85% of businesses expect AI to impact their industry significantly within the next five years [1]. This underscores the need for organizations to adopt AI-driven solutions without delay.
At its core, dynamic process adaptation leverages real-time analysis to improve operations, machine learning for smarter decision-making, and scalable systems to prepare for future demands. To implement this effectively, businesses need to align technical capabilities with their goals. Prioritizing high-quality data, seamless system integration, and workforce readiness is essential to unlocking the full potential of AI automation.
With these key elements in place, companies can confidently take the first steps toward integrating dynamic process adaptation into their operations.
Next Steps for Businesses
To get started, businesses should focus on:
- Reviewing workflows to identify inefficiencies
- Assessing current data management systems
- Pinpointing areas where AI can deliver the most impact
When it comes to implementation, tools like EverEfficientAI can simplify AI integration across various operations. It’s also important to train managers and teams to work effectively with AI systems [1].
For long-term success:
- Track key performance indicators (KPIs) to measure progress
- Regularly update and refine systems
- Invest in ongoing employee training
- Ensure compatibility with tools like Zapier and Make
Tackling challenges like data accuracy and employee training early in the process is crucial. Keep in mind that dynamic process adaptation isn’t a one-time project – it’s a continuous effort to improve and optimize. By committing to this approach, businesses can set themselves up for lasting success in a world increasingly shaped by AI [1][3].
FAQs
What is adaptability in artificial intelligence?
In artificial intelligence, adaptability refers to a system’s ability to adjust its behavior when faced with unexpected changes or challenges. This means the AI can learn in real time, make decisions independently, and respond flexibly to new situations.
For instance, Aisera’s Dynamic Action Flows demonstrate this concept by allowing businesses to automate workflows while staying flexible through real-time orchestration [2]. This capability helps businesses handle market shifts effectively, supporting growth and stability.
When adopting adaptive AI systems, it’s important to focus on smooth integration with existing systems, thorough auditing of AI decisions, and ensuring data accuracy. These steps help organizations take advantage of dynamic process adjustments while maintaining oversight of automated operations.
"Adaptive artificial intelligence is the next generation of AI systems. It can adjust its code for real-world changes, even when the coders didn’t know or anticipate these changes when they wrote the code" [1].
Grasping the concept of adaptability can help businesses better address challenges and make the most of dynamic AI-driven processes, as discussed in this guide.