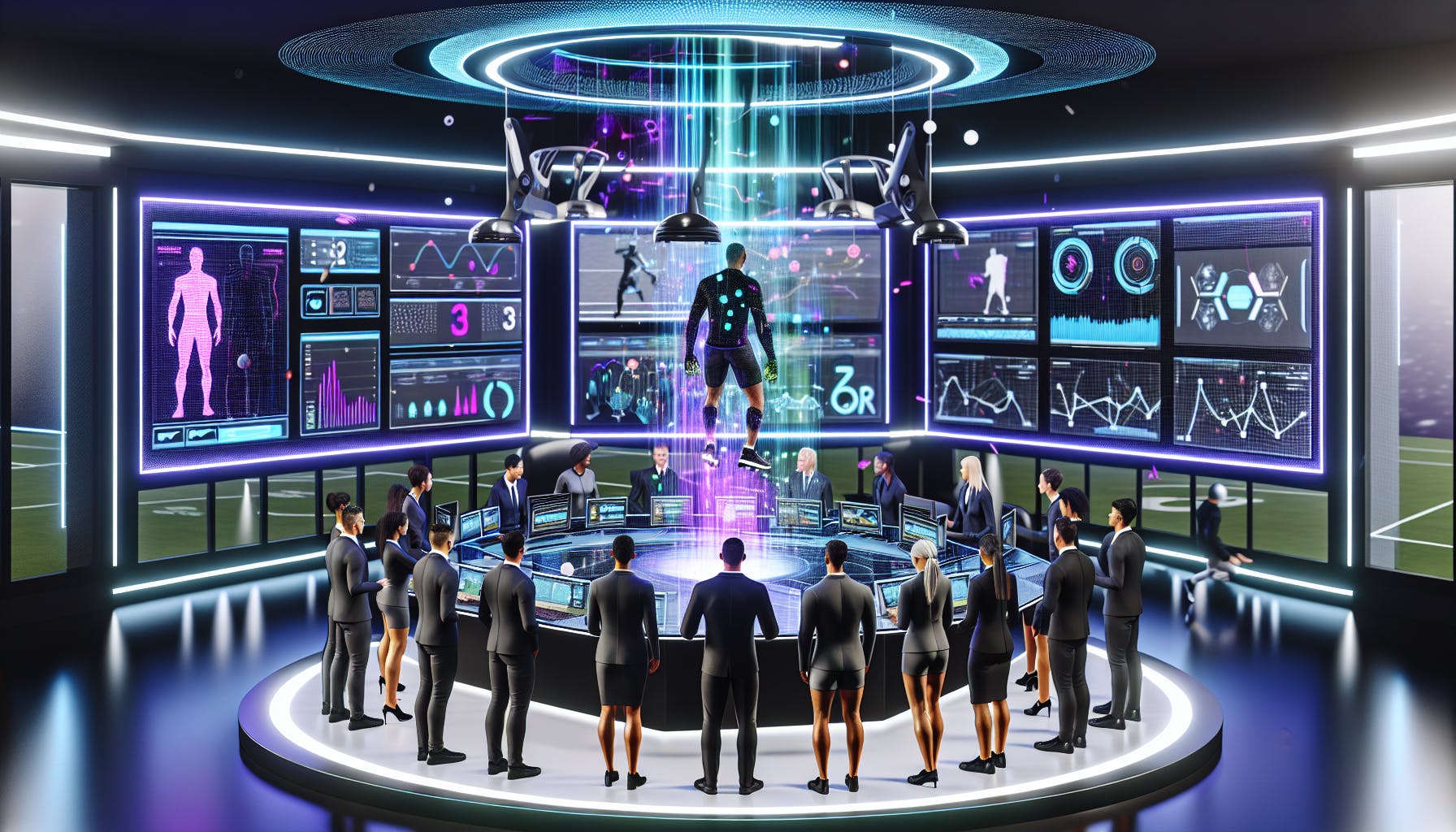
AI in Sports Analytics: Enhancing Team Performance
No doubt, leveraging AI in sports analytics is proving to be a game changer for enhancing team performance.
In this post, you’ll discover how AI and machine learning are revolutionizing sports analytics to unlock transformative advantages – from talent scouting to strategy development.
We’ll explore the key techniques being applied, see real-world examples of AI in action, and anticipate where this technology is heading next to further elevate sports teams.
Introduction to AI in Sports Analytics and Team Performance Monitoring
Overview of AI in Sports Analytics
Sports analytics refers to the analysis of sports performance data to inform decision making and strategy. This can include everything from player statistics to video analysis. Artificial intelligence (AI) and machine learning techniques are being increasingly utilized in sports analytics to uncover deeper insights from data. Common techniques include predictive modeling, computer vision for video analysis, natural language processing (NLP) for analyzing text data, and data visualization. These advanced analytics help coaches and managers better understand factors influencing performance so they can optimize training, strategy, and talent recruitment.
Impact of AI on Team Performance and Strategy Development
AI-powered analytics are transforming how teams monitor and enhance performance across various sports:
- Predictive models forecast player fatigue and injury risk, allowing preventative interventions.
- Computer vision tracks biomechanics to refine techniques and prevent injury.
- NLP mines player interviews to assess morale and inform coaching strategies.
- Analytics dashboards visualize trends in real-time to guide in-game decision making.
By leveraging AI, teams make data-driven decisions to boost on-field performance and strategy development off-field.
Global Sports Analytics Market Trends
The global sports analytics market is projected to grow from $1.9 billion in 2019 to over $5.2 billion by 2024 as teams increasingly adopt advanced analytics solutions. Key drivers include:
- Growing reliance on data-backed decisions for talent recruitment and retention.
- Fans demanding more advanced statistics and insights.
- Wearables and IoT providing richer performance data.
- Cloud computing allowing faster and scalable analytics.
As the market matures, even smaller teams are expected to tap into AI-based analytics.
The Role of Wearable Technology and IoT in Sports Analytics
Wearables like GPS trackers, heart rate monitors, and inertial sensors are widely used to quantify biometrics, movement patterns, and workloads. When integrated with machine learning, these IoT devices provide:
- Real-time fatigue and injury risk monitoring.
- Objective technical and tactical evaluation.
- Automated tracking of performance benchmarks.
This wealth of data will feed the next generation of AI algorithms to further optimize human performance in sports.
How is AI used in sports analysis?
AI is being used in a variety of ways to enhance sports analysis and improve team performance:
Player Monitoring and Injury Prevention
- Wearable devices track biometric data like heart rate, speeds, accelerations, decelerations, and more in real-time during practices and games.
- This data is fed into machine learning algorithms to detect fatigue and injury risk factors for each player individually.
- Coaches can then adjust training loads proactively to prevent overwork and reduce injury.
Game Strategy and Preparation
- Historical play-by-play data, video footage, and scouting reports are synthesized by AI to identify opposing team tendencies and vulnerabilities.
- Coaches can use these AI-generated insights to tailor game plans, run simulations of various strategies, and prepare more effectively.
Skill Development
- Computer vision tracks and quantifies technical skills like shooting mechanics, release timing, footwork, and more for individual players.
- This enables targeted, data-driven skill development programs tailored to each athlete’s specific needs.
In summary, AI and advanced analytics are transforming sports by allowing coaches to monitor athletes, strategize better, and develop skills with more precision – all ultimately aimed at improving performance.
How is AI used in analytics?
AI is transforming sports analytics in several key ways:
Player Performance Tracking
Wearable devices and computer vision systems allow for advanced tracking of player movements, biometric data, and technique. This provides coaches and analysts with incredibly detailed data to evaluate performance, fitness, injury risk and areas for improvement. Common use cases include:
- Tracking player speed, acceleration, deceleration, change of direction, jumps using IMU sensors and GPS. This allows detailed load monitoring.
- Tracking technical skills like shooting mechanics in basketball, swing mechanics in golf/baseball using computer vision. Enables remote coaching.
- Predicting injury risk by detecting changes in movement patterns early. Critical for injury prevention.
Advanced Statistics and Modeling
AI and machine learning unlock next-level analytics and models for gaining strategic advantage:
- Predicting the probability of different plays and outcomes using historical play-by-play data. Enables better planning.
- Determining strengths/weaknesses of opponents through analysis of past games. Critical for matchup strategy.
- Optimizing lineups and rotations based on players’ attributes and opposition. Maximizes winning probability.
Cognitive Highlights and Pattern Recognition
Automated analysis of video footage using CV/NLP provides tactical insights:
- Generating key highlights and tactical review clips from game footage using CV and summarization algorithms. Saves coaching staff hours of manual analysis.
- Detecting patterns and tendencies of opponents over time. Uncovers strategic weaknesses to exploit.
- Analyzing emotional state and communication of players/coaches. Provides psychological and motivational insights.
The applications of AI in sports analytics are rapidly evolving. With more data than ever before, AI promises to unlock unprecedented performance gains for teams willing to embrace a data-driven approach.
How is this AI being used in the sport event industry?
AI technologies are being utilized across professional sports teams and leagues to enhance performance monitoring, medical care, officiating, front office operations, and more.
Some examples include:
-
Player performance tracking: Wearable devices and computer vision systems track player movements, vitals, and biometrics during games and practices. This data feeds into predictive models that optimize training routines, reduce injury risk, and inform coaching decisions.
-
Officiating automation: AI video analysis tools help referees make more accurate calls in real-time by detecting fouls and close plays automatically. This improves game flow and fairness.
-
Medical analytics: Algorithms analyze player medical histories, vitals data, and imaging scans to diagnose injuries earlier and recommend preventative interventions. This accelerates return-to-play timelines.
-
Front office analytics: Predictive analytics on player performance, salary caps, fan preferences and ticketing data help team managers make smarter roster decisions, set ticket prices, and optimize stadium operations.
By integrating AI across the entire sports organization, teams and leagues are enhancing performance, medical care, and operational efficiency. As the technology continues improving, its applications in sports will only expand further.
sbb-itb-178b8fe
What is the future of artificial intelligence in sports?
Artificial intelligence is rapidly transforming the world of sports. AI and machine learning offer new ways to analyze performance, gain insights, and enhance training. As the global sports analytics market grows, AI is becoming indispensable.
Some key ways AI will shape the future of sports:
-
Enhanced analytics and insights: AI can process huge volumes of data from sensors and video to provide granular performance analysis. This allows identifying strengths, weaknesses and opportunities for improvement.
-
Injury prediction and prevention: By analyzing biomechanics and load management using AI and IoT devices, teams can better predict and help prevent injuries. This improves career longevity.
-
Computer vision for officiating: Computer vision AI can augment human referees and officials by providing more objective decision making in real-time during matches. This increases fairness and accuracy.
-
Generative coaching: AI techniques like NLP can generate personalized training plans, tailored feedback, and coaching advice for athletes. This makes coaching more accessible.
-
Immersive experiences: Generative AI will create more engaging, customized media coverage, social sharing, fantasy sports, and gaming experiences for fans.
As costs fall, AI and ML will become indispensable for amateurs and pros alike. Beginners can gain a competitive edge by embracing AI early, while top teams integrate it holistically. AI promises to make sports fairer, safer and more engaging for all.
Key AI-ML Techniques in Sports Analytics
Machine Learning for Predictive Analytics in Talent Scouting
Machine learning models can analyze player statistics and gameplay video to identify patterns and make predictions about future performance. This allows teams to more accurately evaluate talent and potential contributions to the team. Common use cases include:
- Predicting injury risk based on biomechanics and workload
- Forecasting player development arcs over multi-year periods
- Evaluating skills and attributes that indicate success in a sport
- Automating video analysis for scouting reports
By leveraging predictive analytics, teams can optimize their talent scouting and acquisition processes.
Enhancing Sports Training with Computer Vision
Applying computer vision techniques like pose estimation and object tracking during practices allows granular analysis of mechanics, movement patterns and workloads. This video-based analysis helps coaches:
- Detect weaknesses in technique or imbalance in strength
- Compare workout volume across athletes
- Customize training programs to target specific skills
- Track return-to-play progress after injuries
Optimized training routines based on computer vision analytics can directly improve player performance.
Cognitive and NLP Technologies in Sports Media Analysis
Natural language processing tools can ingest online news, social media conversations and fan forums to extract sentiment, themes and narrative arcs. This allows teams to:
- Benchmark brand health and affinity
- Identify PR pain points to improve messaging
- React quicker to emerging controversies
- Cultivate fan engagement and loyalty
By keeping a pulse on fan discussions, cognitive analytics empowers more audience-centric communications.
Data Visualization Techniques for Strategy Development
Interactive data visualizations help extract insights from player tracking data, health metrics and advanced scouting analytics. Useful implementations include:
- Identifying matchup advantages against opponents
- Determining optimal lineups and rotations
- Pinpointing areas needing tactical adjustments
- Conveying analytics findings to stakeholders
Dataviz enables data-driven decision making for competitive strategy formulation.
Examples of AI in Sports Analytics and Team Performance
AI in Sports Training: Case Study on Load Management
The Los Angeles Lakers partnered with a sports science company to leverage AI and wearable technology for injury prevention. Players wore biometric jerseys during practices to track workload, fatigue levels, heart rate variability and other metrics. By applying machine learning algorithms to this data, the training staff gained more precision in planning rest days and regulating training intensity to optimize player availability over a grueling 82-game regular season plus playoffs.
Computer Vision in Action: Automated Video Analysis for Football
A top European football club deployed computer vision and natural language processing to automatically index and tag video footage from practices and matches. Coaches can now swiftly review clips by player, play type, result and other filters rather than manually combing through hours of footage. This accelerated the feedback cycle and enabled quicker adjustments to strategy based on recent performance.
Deep Learning for Fan Engagement and Live Match Updates
A popular cricket app tapped into computer vision and natural language generation to provide personalized, real-time match updates to fans. Computer vision interprets live video feeds to track ball-by-ball events. Natural language algorithms then generate text commentary and graphics tailored to each fan’s declared favorite players and teams. This level of personalization drives engagement.
Generative AI in Sports: Revolutionizing Content Creation
AI-generated content is gaining traction in sports media. Algorithms can churn out draft articles on recurring events like post-game analyses or tournament previews. Natural language generation has also shown promise for crafting personalized fan experiences. A football club experimented with AI-generated welcome packets for new season ticket holders that wove in details on the purchaser’s hometown and past team support. Though human review is still required, AI promises to scale content production.
Strategic Implementation of AI in Sports Analytics
Assessing Organizational Readiness for AI-ML Integration
Integrating AI and machine learning into sports analytics requires careful planning and assessment of an organization’s data infrastructure, processes, and personnel skills. Best practices include:
- Auditing existing data sources, data pipelines, and analytics tools to identify gaps or blockers for AI implementation
- Assessing in-house data science skills and determining if additional hiring or training is needed
- Reviewing current analytics workflows and identifying areas that can be enhanced with AI automation
- Prioritizing 1-2 pilot AI projects with clear business impact, starting small to demonstrate ROI
Overcoming Key Challenges in Sports Analytics AI Deployment
Common hurdles when deploying AI in sports analytics include:
- Model accuracy and overfitting due to limited datasets
- Organizational resistance or lack of alignment on AI adoption
- Poor change management when transitioning from traditional analytics to AI
Proven methods to address these issues include:
- Leveraging techniques like transfer learning to improve model accuracy
- Educating stakeholders on AI benefits and risks to gain buy-in
- Phased rollout focusing on augmenting rather than replacing existing analytics
Decision Making: In-House Development vs. Sports Analytics Platforms
Key considerations when deciding between custom in-house AI development versus leveraging third-party platforms:
In-House Development
- More control and customization for proprietary models
- Ability to closely integrate AI with internal data and systems
- Higher costs for data science team and infrastructure
Third-Party Platforms
- Faster time-to-value by building on existing AI models
- Lower costs by leveraging shared cloud infrastructure
- Less control or customization for unique team needs
A hybrid approach combining in-house AI skills with third-party platforms can provide the best of both worlds.
Ensuring Ethical Use of AI and Data Privacy in Sports
It’s critical to consider ethics and privacy when using AI for analytics:
- Conduct algorithm audits to detect potential model bias
- Anonymize player data or obtain explicit consent for AI model training
- Provide transparency to stakeholders on how AI models are used
- Develop guidelines for ethical use of predictions from AI systems
The Future of AI in Enhancing Sports Team Performance
Evolving Data Analytics in Real-Time Decision Making
Advances in connectivity and exponential data growth are enabling sports teams to utilize predictive and prescriptive analytics to inform decision making in real-time. With sensors and wearable technology generating vast datasets, machine learning models can identify patterns and make recommendations on substitutions, formations, plays etc. This allows coaches to leverage data-driven insights during matches.
However, real-time analytics comes with challenges like dealing with noise in sensor data and lag in computational analysis. Edge computing solutions may help by allowing some processing on local devices. Overall, expect analytics to become increasingly dynamic.
Generative AI for Dynamic Strategy Development
Experiments are underway in using large language models to generate content and adjust in-game strategy. For instance, systems could analyze match data and produce written briefings on opposition weaknesses to exploit. Or provide personalized scouting reports on upcoming opponents.
Going further, AI could even suggest specific plays and tactics optimized for player strengths against a given opponent. This could enable more adaptive and optimized game plans tailored to each match.
Scaling from Incremental Improvements to Transformative Advantages
Currently most teams use analytics in siloed applications, leading to incremental optimizations. But as predictive modeling and computational power improves, expect more integrated and automated analytics pipelines.
Soon AI may transition from providing piecemeal inputs to enabling a pervasive, data-driven "culture of optimization" empowering transformative leaps in performance. Teams could realize compounding competitive advantages as analytics shifts from niche tools to an organization-wide enabler.
Anticipating the Impact of AI on the Global Sports Analytics Market
As AI capabilities grow, demand for analytics solutions will likely dramatically rise across teams and leagues looking to gain an edge. This could expand the market size and disrupt the competitive landscape.
First movers with proprietary algorithms and datasets may build hard-to-match advantages. Expect consolidation too as top analytics firms incorporate AI into turnkey platforms and gain market share through superior insights. The democratization of basic analytics will continue, but truly game-changing offerings will remain rare and valuable.
Related posts
- AI in Healthcare: Bridging the Efficiency Gap
- AI and Automation: Navigating the Future of Efficiency
- AI in Business: Streamlining Operations for Efficiency
- AI for Human Resources: Predictive Analytics for Strategic Planning